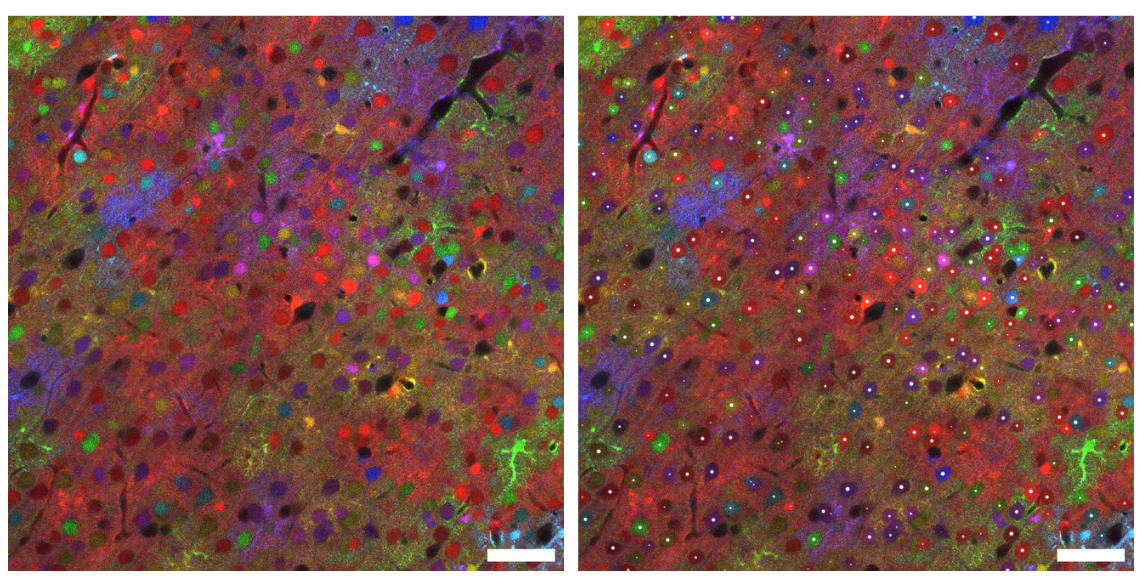
An international team of researchers led by Ignacio Arganda (University of the Basque Country — UPV/EHU, Ikerbasque, Donostia International Physics Center, and Biofisika Institute) and Arrate Muñoz Barrutia (Carlos III University of Madrid, Gregorio Marañón Health Research Institute) has developed BiaPy, an open-source artificial intelligence platform that facilitates the analysis of biomedical images using deep learning techniques. The work has been published in the prestigious journal Nature Methods.
Image analysis is an essential tool in biomedicine, used to study cellular structures, tissues, and organs across multiple disciplines. However, applying artificial intelligence to analyze these images has traditionally been reserved for experts in programming and data science. BiaPy breaks this barrier by offering an easy-to-use platform that enables the application of advanced AI models without requiring specialized technical knowledge.
“The goal of BiaPy is to democratize access to artificial intelligence in bioimaging, allowing more scientists and healthcare professionals to harness its potential without needing advanced knowledge in programming or machine learning,” explains Daniel Franco, first author of the study and currently a postdoctoral researcher at the MRC Laboratory of Molecular Biology and the University of Cambridge (United Kingdom).
BiaPy enables various types of scientific image analysis, such as automatically identifying cells or other biological structures, counting elements, classifying samples based on appearance, or enhancing image quality to reveal finer details. These analyses can be performed on both two-dimensional images and three-dimensional datasets obtained through different microscopy techniques. Moreover, BiaPy is designed to be efficient and scalable: it can handle a wide range of data volumes, from a few small images to terabytes of information, such as those generated when scanning entire tissues or organs.
The tool relies on the use of "artificial intelligence models" — algorithms trained to recognize patterns in images, similarly to how the human eye identifies shapes or colors. To create a model, it is first trained with examples — for instance, images where cells have already been manually annotated. With sufficient training, the model can then perform these tasks automatically, even on new, previously unseen images.
"Additionally, BiaPy is integrated into the BioImage Model Zoo (bioimage.io), a database where researchers worldwide share pre-trained models. Thanks to this integration, BiaPy users can easily reuse existing models for new images or train their own models," explains Arrate Muñoz, senior co-author of the study and a member of the European AI4Life consortium that developed the BioImage Model Zoo.
This tool is already being used in advanced scientific projects. One example is CartoCell, a software solution developed in collaboration with the laboratory led by Luis M. Escudero (Institute of Biomedicine of Seville — Hospital Universitario Virgen del Rocío/CSIC/University of Seville). CartoCell analyzes microscopy images to reveal hidden patterns in the shape and distribution of cells within 3D epithelial tissues from different organisms.
Another notable case is its application in collaboration with the laboratories of Emmanuel Beaurepaire (École Polytechnique, France) and Jean Livet (Institut de la Vision, Paris). These groups have developed a microscopy technique called ChroMS, which produces enormous three-dimensional images of entire brains using fluorescent colors generated by jellyfish and coral proteins. BiaPy is used to automatically detect each cell in these large-scale images, even in densely populated areas of the brain, enabling the study of brain development by reconstructing the lineage of cells based on their colors and three-dimensional positions.
As an open-access tool, BiaPy is freely available to the scientific community, encouraging collaboration and continuous software improvement. It can be used on personal computers, servers with multiple graphics cards, or cloud computing environments. Its easy installation ensures that experiments can be easily reproduced across different setups, promoting open and reproducible science.
In the words of Ignacio Arganda, senior author of the work, "The development of BiaPy represents an important step toward democratizing advanced computer vision in microscopy. Its accessible design and focus on open collaboration lower technical barriers, enabling more researchers and healthcare professionals to apply computer vision in their studies. Its compatibility with various computing environments and open-source nature make it a platform with great potential to drive innovation and accelerate scientific discovery."
For more information about BiaPy, visit:
http://biapyx.github.io/
https://www.biorxiv.org/content/10.1101/2024.02.03.576026v3.abstract
Bibliographic eference:
Daniel Franco-Barranco, Jesús A. Andrés-San Román, Ivan Hidalgo-Cenalmor, Lenka Backová, Aitor González-Marfil, Clément Caporal, Anatole Chessel, Pedro Gómez-Gálvez, Luis M. Escudero, Donglai Wei, Arrate Muñoz-Barrutia & Ignacio Arganda-Carreras
BiaPy: accessible deep learning on bioimages
Nature Methods, Volume 22, No. 4, 2025.
DOI: https://doi.org/10.1038/s41592-025-02699-y